I. Data-Driven (Machine Learning-based, Model-free Approaches) for Nonlinear and Large-Scale Control
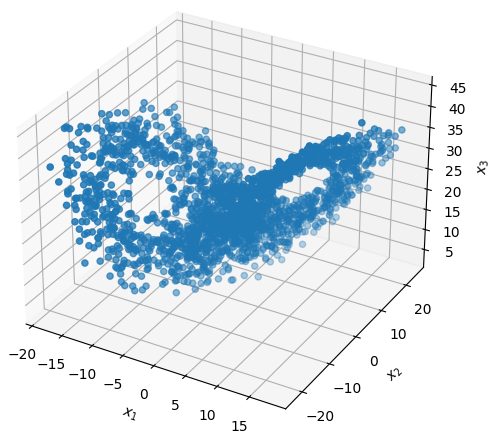
Chemical processes are distinctively challenging to control with outstanding performance. Nonlinearity, uncertainty, and large scale are often intertwined, which exacerbates the complexity of process control problems. For the current chemical process industry that is pursuing not only higher efficiency but also sustainability, flexibility, and modularity, the role of process automation and control should not be underestimated.
The emergence of data-driven control based on machine learning techniques bring new opportunities and perspectives that pushes the limit of our capacity to resolve process complexities. In the meantime, they draw our attention to fundamental issues in control and call for extensive effort to integrate process control with the contemporary advances in machine learning.
Theoretical Directions
- Data-driven approaches for state observation for nonlinear systems
- Data-driven approaches for state-space dynamical analysis for nonlinear systems
- Learning approaches for the dissipative/passive properties of nonlinear systems
- Controller synthesis and analysis for large-scale interconnected systems
- Reliable machine learning for control-theoretic problems
- Fundamental advantage over classical model-based control
Examples of Potential Applications
- Multiphase reactors -- Bubble columns, trickle beds, fluidized beds
- Energy systems -- Integrated HVAC systems, smart grids, wind farms
- Advanced manufacturing -- 3D printing, biopharmaceutical processes
II. Structured and Scalable Algorithms for Black-Box Optimization
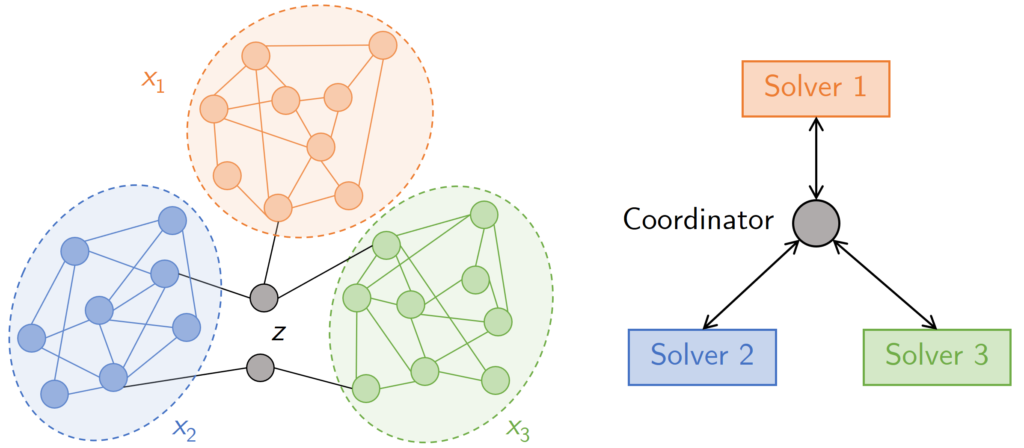
Decision making in environments where a transparent model with algebraic expressions is unavailable poses significant challenge to typical mathematical optimization methods that are based on easily accessible derivative information. Such problems, arising from a multitude of computational science and engineering fields, need to be solved in an efficient way through parsimonious sampling and surrogate modeling. The consideration of first principles can also be of decisive importance.
Black-box optimization are typically restricted to low-dimensional and unstructured problems, and its convergence behavior, especially for global optimization and cases with discrete decisions, is often unsatisfactory. These challenges motivate more comprehensive approaches towards successful applications to real-world engineering problems.
Theoretical Directions
- Large-scale and distributed optimization algorithms
- Optimization on manifolds or spaces of structured data
- Relation between dynamical systems theory and optimization
Examples of Potential Applications
- Molecular discovery: Finding active compounds using database and automated experimentation
- Process and product design: Integrated optimization based on multiscale simulations
- Hyperparameter optimization: Coupled algorithm tuning, transfer learning
III. Collaborative Projects
The boundary of process systems engineering has been and is continually being extended by new technologies that improve our capability of sensing, actuation, computation and enlarge the scope of engineering activities. Our group conducts interdisciplinary research through collaborations. We currently collaborate with
- Prof. Lilian Hsiao on control of nanomanufacturing processes, and
- Profs. Milad Abolhasani, Joshua Pierce, Caroline Proulx, & Melanie Simpson on self-driving laboratories for molecular discovery.
As a new and expanding research group, potential directions for collaborative research are always being actively explored.
The contents on this webpage was updated on August 18, 2024.